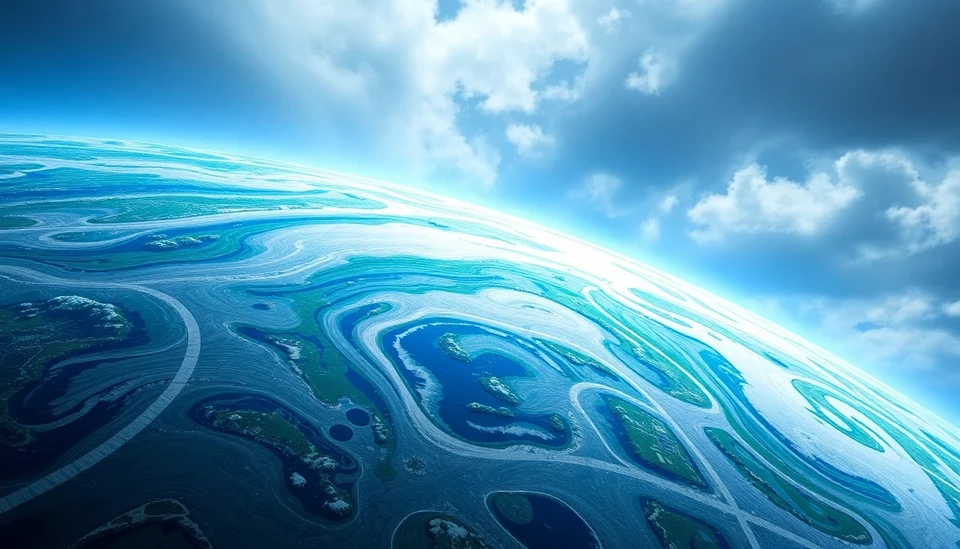
The landscape of weather forecasting is rapidly transforming as artificial intelligence (AI) technologies emerge as powerful tools for generating hyper-local, highly accurate weather predictions. This article explores the advancements in AI-driven weather models that promise to take meteorological forecasting to unprecedented levels of detail and precision.
Traditionally, weather forecasts have relied on a combination of historical data, satellite imagery, and physical models. However, the shift towards AI represents a significant pivot in forecasting methodologies, utilizing machine learning algorithms that can analyze vast datasets quickly and effectively. These algorithms are designed not just to predict basic weather conditions but to provide granular insights that can potentially change how industries prepare for severe weather events.
A standout feature of this emerging technology is its ability to deliver hyper-local forecasts, making predictions that are incredibly specific in terms of location and time. For instance, while conventional forecasts may provide generalized weather conditions for a broader area or region, AI models can analyze localized data to predict rainfall patterns or wind gusts for specific neighborhoods or even individual streets.
This enhanced level of detail is particularly valuable for sectors that are heavily impacted by weather, such as agriculture, construction, and emergency services. Farmers can rely on AI forecasts to determine the optimal times for planting or harvesting crops, while construction teams can schedule work with higher efficiency by anticipating adverse weather conditions. Likewise, emergency services can better allocate resources and plan for disaster response by receiving advanced warnings of severe weather events.
Industry leaders and meteorologists are enthusiastic about the potential of these AI technologies. They herald the application of machine learning for processing vast troves of meteorological data, including real-time weather information and historical weather patterns, allowing for simulations and predictions that were previously unfeasible. Additionally, these models can adapt and improve over time, learning from new data and changing weather patterns, thus continually enhancing their predictive capabilities.
While the prospects are promising, there remain challenges to overcome. AI-driven models require extensive datasets and significant computational power to function effectively. Furthermore, there are ongoing discussions within the meteorological community about the need for transparency in AI processes, particularly regarding the risks of over-reliance on these models and ensuring that forecasters interpret the results within proper contexts.
This revolution in weather forecasting is just beginning, but it is poised to change how information is communicated to the public. Improvements in user interfaces and accessibility mean that detailed, granular forecasts may soon be available at the fingertips of individuals and businesses alike, facilitating a proactive rather than reactive approach to weather-related challenges.
As we look ahead, the integration of AI into weather forecasting could very well redefine our relationship with the elements, paving the way for smarter decisions and enhanced safety in weather-dependent activities.
With this advancement, the future of weather forecasting looks not only more accurate but also deeply personalized, further closing the gap between meteorologists and the general public.
#WeatherForecasting #AIModels #HyperlocalPredictions #Meteorology #ArtificialIntelligence #WeatherTechnology #GranularForecasts
Author: Megan Clarke